PU Shortages and Cloud Costs Impact AI
In the rapidly evolving landscape of artificial intelligence (AI), the interplay between processing unit (PU) shortages and escalating cloud costs has emerged as a critical challenge for researchers, developers, and organizations alike. As the demand for computational power continues to surge, the scarcity of PUs and the mounting expenses associated with cloud infrastructure are significantly impacting AI projects. This article delves into the intricate web of challenges posed by PU shortages and rising cloud costs in the realm of AI, exploring their implications, strategies for mitigation, and the latest innovations driving solutions for a more sustainable future in AI development.
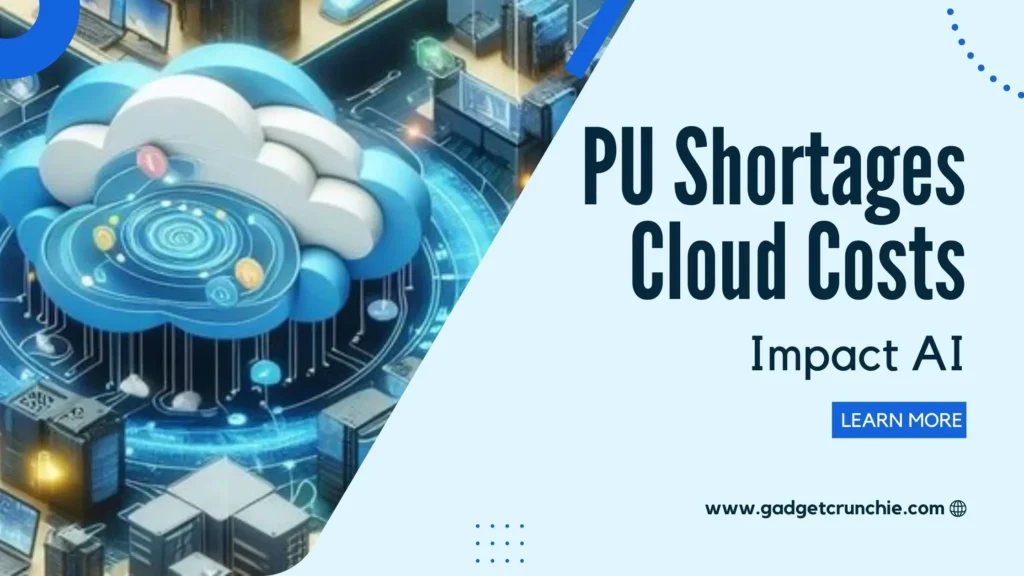
1. Introduction to the Challenges of PU Shortages and Cloud Costs in AI
Exploring the Increasing Demand for Processing Units in AI
In the world of artificial intelligence (AI), the hunger for processing units (PUs) seems insatiable. As AI applications become more sophisticated and data-hungry, the demand for PUs continues to rise. But with shortages looming, can AI keep up with its own appetite?
Understanding the Impact of Cloud Infrastructure on AI Development
Cloud computing has been a game-changer for AI development, offering scalable resources and flexibility. However, as the reliance on cloud infrastructure grows, so do the costs. How are these escalating expenses affecting the landscape of AI projects?
2. The Impact of PU Shortages on AI Development
Current Status of Processing Unit Shortages and Their Effects on AI Projects
The scarcity of PUs is casting a shadow over AI development. From delays in project timelines to increased competition for limited resources, the impact of shortages is palpable. How are AI projects coping in the face of this challenge?
Challenges Faced by AI Researchers and Developers Due to PU Shortages
For AI researchers and developers, navigating the PU shortage landscape is akin to a high-stakes game of musical chairs. How are they adapting to this new reality, and what strategies are proving effective in overcoming these obstacles?
3. Rising Cloud Costs and Their Influence on AI Projects
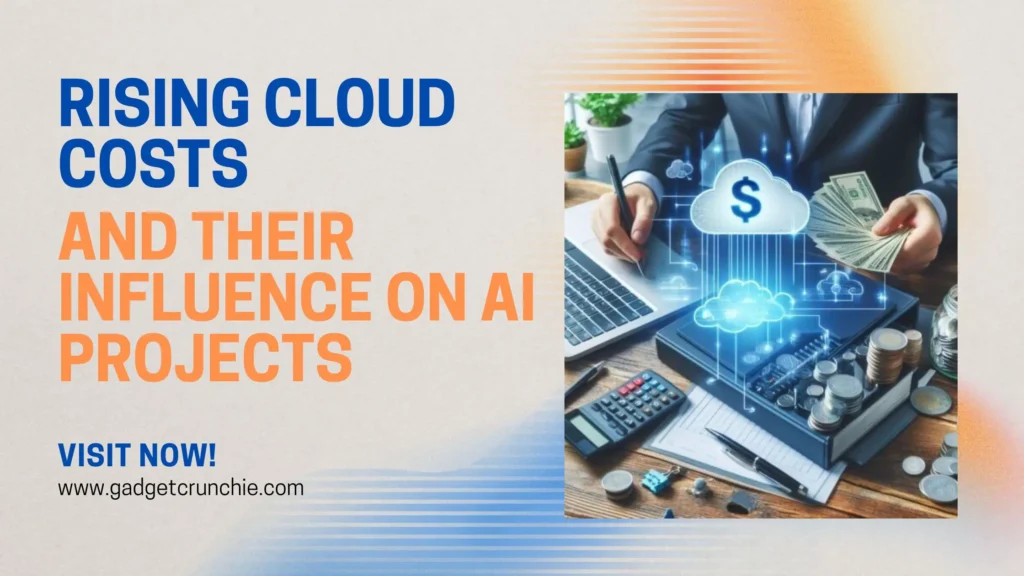
Factors Contributing to Escalating Cloud Costs for AI Workloads
As AI workloads expand and become more complex, so do the bills from cloud service providers. What factors are driving up costs, and how are organizations grappling with this financial burden?
Effects of Rising Cloud Costs on the Viability of AI Initiatives
In a world where innovation often hinges on budget constraints, the soaring cloud costs are putting a strain on the viability of AI initiatives. How are organizations recalibrating their strategies to ensure their AI projects remain sustainable in the long run?
4. Strategies for Mitigating PU Shortages in AI Applications
Utilizing Alternative Processing Solutions to Counter PU Shortages
In the face of PU shortages, AI practitioners use alternative processing solutions to keep their projects afloat. What are the options available from GPUs to FPGAs, and how effective are they in bridging the PU gap?
Implementing Efficient Resource Allocation Techniques to Manage PU Constraints
To thrive in a world of limited PUs, efficient resource allocation is key. How are AI teams optimizing their workflows, leveraging resources judiciously, and finding innovative ways to circumvent PU constraints?
5. Optimizing Cloud Spending for AI Workloads
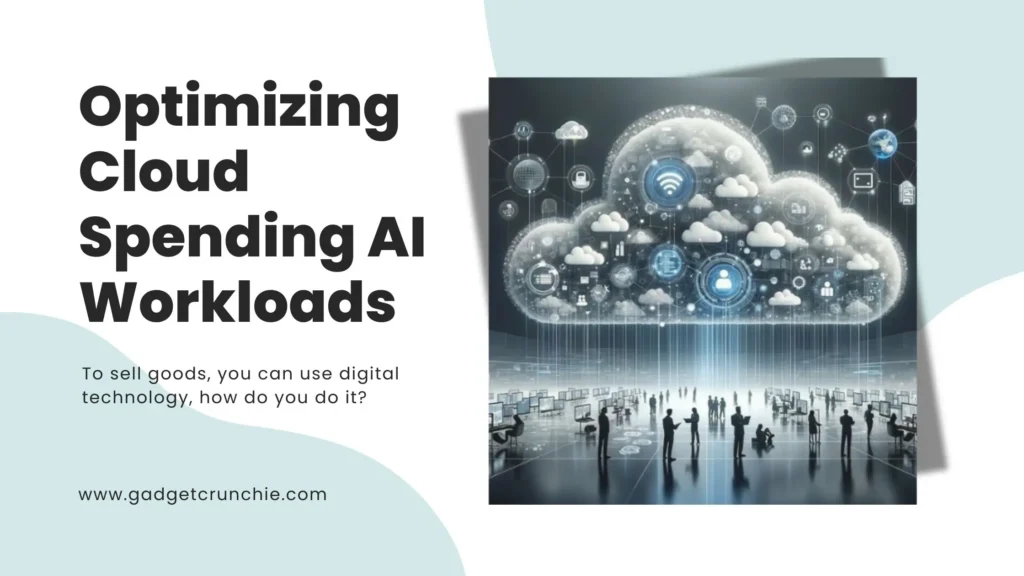
Best Practices for Cost-Effective Cloud Resource Allocation in AI Projects
When it comes to AI projects, optimizing cloud spending is key to keeping costs under control. Best practices include rightsizing instances, utilizing spot instances for non-time-sensitive workloads, and implementing auto-scaling to adjust resources based on demand. By closely monitoring usage and adopting a pay-as-you-go approach, organizations can ensure that cloud resources are allocated efficiently for AI workloads.
Leveraging Cloud Cost Management Tools for AI Development Efficiency
To streamline cloud cost management for AI development, leveraging tools like AWS Cost Explorer, Google Cloud Cost Management, or Azure Cost Management can provide valuable insights into resource usage and cost allocation. These tools can help identify cost-saving opportunities, track spending trends, and optimize resource utilization, ultimately promoting efficiency and cost-effectiveness in AI projects.
6. Innovations in AI Hardware to Address PU Shortages
Emerging Technologies and Hardware Solutions to Alleviate Processing Unit Scarcity
In response to the ongoing PU shortages, innovative hardware solutions are being developed to address the scarcity of processing units in AI applications. Technologies such as neuromorphic chips, quantum computing, and customized AI accelerators are paving the way for more efficient and powerful AI hardware, offering alternative solutions to traditional processing units.
Impact of AI-Specific Hardware Developments on Mitigating PU Shortages
The advancements in AI-specific hardware developments not only help alleviate PU shortages but also contribute to enhancing AI performance and efficiency. By leveraging specialized hardware optimized for AI workloads, organizations can circumvent the limitations posed by processing unit scarcity, unlocking new possibilities for more robust and scalable AI applications.
7. Future Trends and Solutions for Managing PU Shortages and Cloud Costs in AI
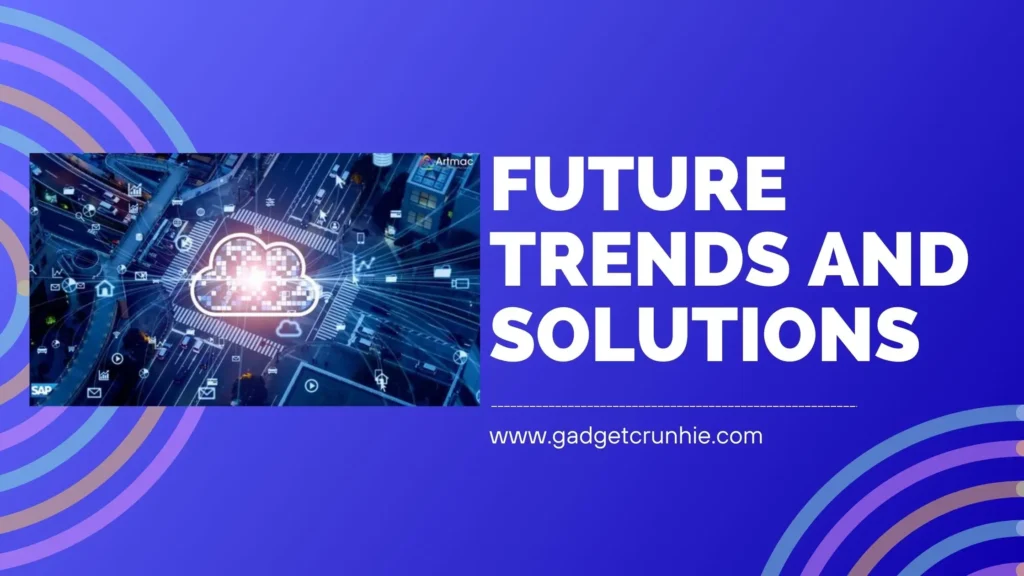
Predictions for the Evolution of PU Availability and Cloud Cost Trends in AI
Looking ahead, the future of AI development will likely see improvements in PU availability and advancements in cloud cost management strategies. As technology continues to evolve, we can expect a more diverse range of processing units and cost-effective cloud solutions to support the growing demands of AI applications.
Innovative Approaches and Technological Advances for Sustainable AI Development in the Face of PU Shortages and Rising Cloud Costs
To address the challenges posed by PU shortages and rising cloud costs, innovative approaches such as edge computing, federated learning, and AI model compression techniques are emerging as sustainable solutions for AI development. By leveraging these technological advances and adopting a holistic approach to resource management, organizations can navigate the complexities of PU shortages and cloud costs while driving continuous innovation in the field of AI.In conclusion, navigating the complex terrain of PU shortages and cloud costs in AI requires a multifaceted approach that combines technological innovation, strategic resource allocation, and forward-thinking solutions. By staying abreast of emerging trends, leveraging cost-effective strategies, and embracing advancements in AI hardware, individuals and organizations can overcome these hurdles and propel the field of artificial intelligence towards continued growth and success. Embracing resilience and adaptability in the face of challenges, the AI community is poised to unlock new possibilities and drive impactful advancements in the realm of intelligent technologies.