In the rapidly evolving landscape of artificial intelligence (AI), the concepts of trust, risk, and security management play a crucial role in ensuring the responsible and effective deployment of AI technologies. This article delves into the realm of AI Trust, Risk, and Security Management (TRiSM), exploring the significance of building trust in AI systems, identifying potential risks associated with AI deployment, and implementing robust security measures. By examining strategies for fostering trust, addressing ethical considerations, navigating compliance frameworks, and anticipating future challenges, this article aims to provide a comprehensive overview of the key considerations in managing trust, risk, and security in the realm of AI.
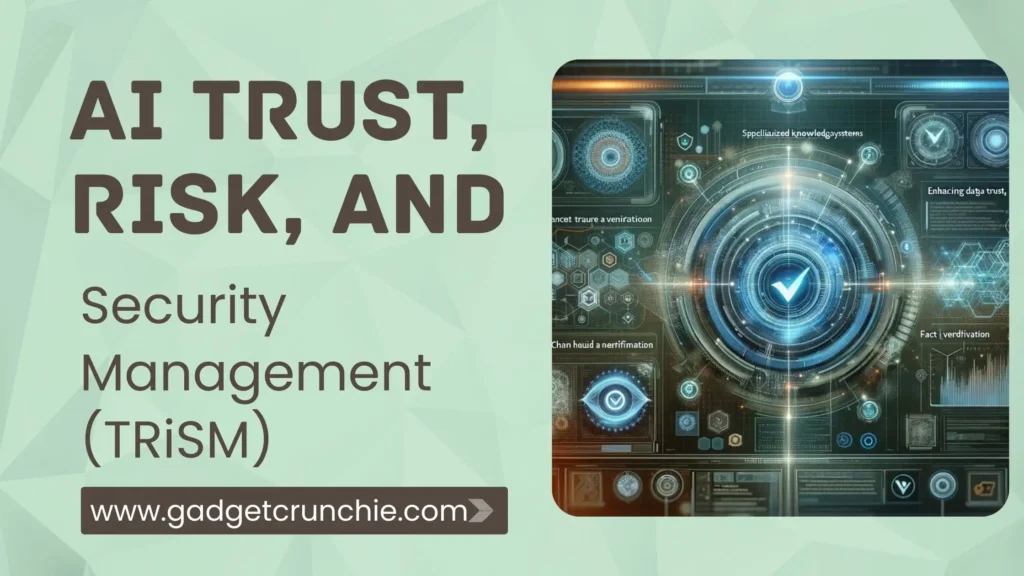
Introduction to AI Trust, Risk, and Security Management (TRiSM)
In this dynamic technological landscape, the convergence of artificial intelligence and cybersecurity has given rise to AI Trust, Risk, and Security Management (TRiSM). This framework aims to address the evolving challenges associated with ensuring the trustworthiness, managing risks, and enhancing security in AI systems.
Defining AI Trust, Risk, and Security Management
AI Trust, Risk, and Security Management (TRiSM) encompass the strategies, processes, and practices employed to foster trust, identify risks, and implement security measures in artificial intelligence systems. By integrating these components, organizations can navigate the intricate landscape of AI technologies more effectively and responsibly.
Understanding the Importance of Trust in AI Systems
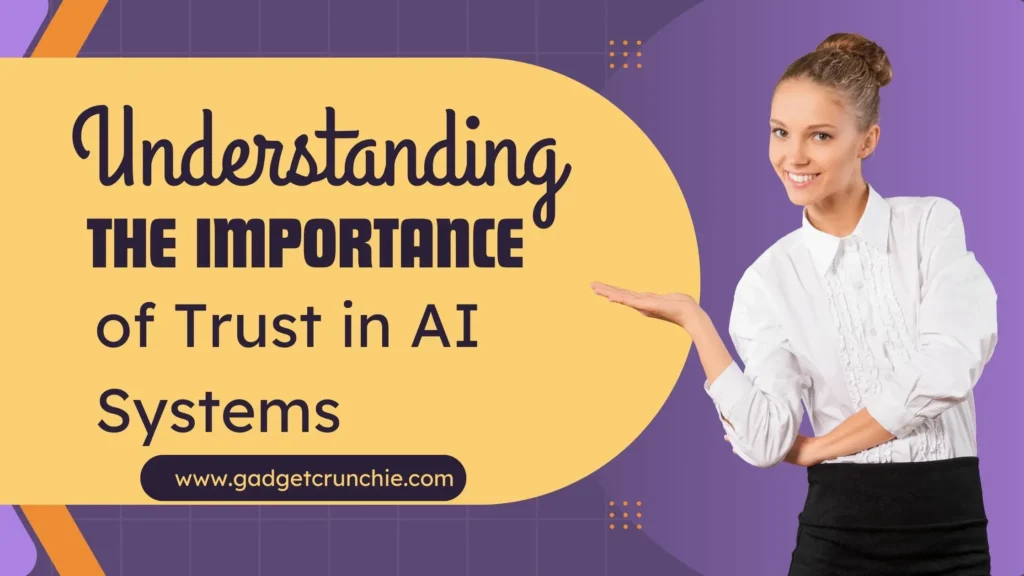
Trust forms the foundation of successful AI implementations, influencing user acceptance, reliability, and societal impact. Building and maintaining trust in AI technologies is crucial for fostering positive relationships between humans and machines, driving innovation, and ensuring ethical decision-making.
Building Trust in AI Technologies
Establishing trust in AI systems requires transparency, accountability, and ethical considerations throughout the development lifecycle. By promoting explainability, fairness, and user-centric design, organizations can enhance the credibility and acceptance of their AI solutions among stakeholders.
Impacts of Trust on AI Adoption
The level of trust in AI systems directly influences adoption rates, customer satisfaction, and regulatory compliance. Organizations that prioritize trust-building efforts can differentiate themselves in the market, build stronger relationships with users, and mitigate potential risks associated with AI deployment.
Identifying Risks Associated with AI Deployment
While AI offers numerous benefits, it also introduces inherent risks that organizations must address to ensure successful implementation and operation. Understanding and proactively managing these risks are essential for maximizing the value of AI investments and safeguarding against potential pitfalls.
Common Risks in AI Implementation
From biased decision-making to data privacy concerns, organizations face a range of risks when deploying AI systems. Issues such as algorithmic bias, model vulnerabilities, and compliance gaps can undermine the trustworthiness and effectiveness of AI solutions, leading to reputational damage and financial losses.
Risk Assessment and Mitigation Strategies
Conducting comprehensive risk assessments and implementing robust mitigation strategies are critical components of effective AI risk management. By identifying potential threats, vulnerabilities, and compliance issues early on, organizations can proactively address challenges, enhance resilience, and optimize the performance of their AI systems.
Implementing Security Measures in AI Systems
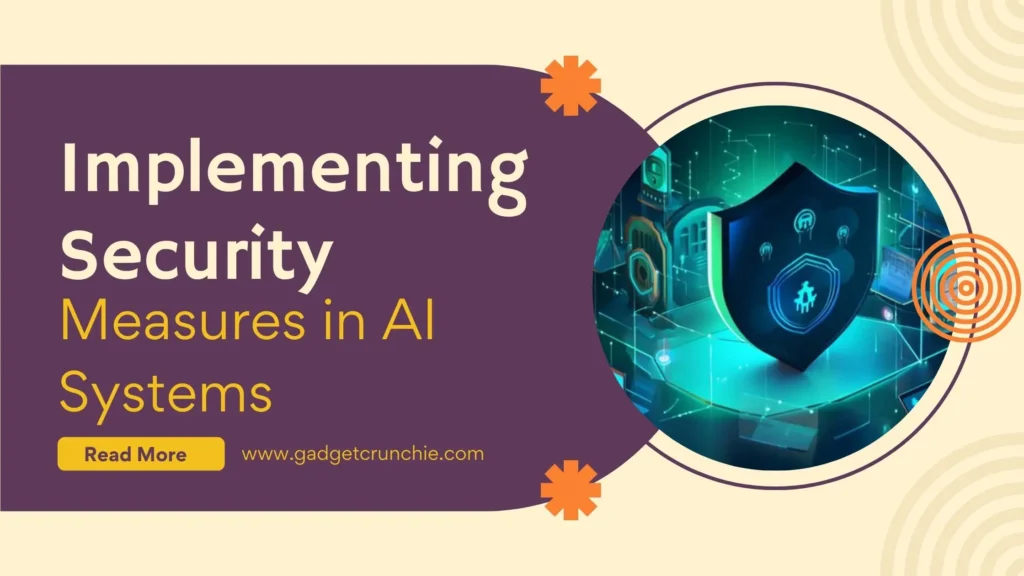
Security remains a top priority in AI development, as the proliferation of connected devices and data exchange channels introduces new attack vectors and vulnerabilities. By integrating security measures into AI systems from the outset, organizations can safeguard against cyber threats, protect sensitive information, and uphold the integrity of their operations.
Security Challenges in AI Development
Securing AI systems presents unique challenges, including safeguarding data integrity, preventing adversarial attacks, and ensuring regulatory compliance. Organizations must address these challenges by implementing encryption protocols, access controls, and threat detection mechanisms to fortify the security posture of their AI environments.
Best Practices for Securing AI Systems
Adopting best practices for securing AI systems involves a multi-faceted approach that encompasses technical, organizational, and regulatory aspects. By adhering to security frameworks, conducting regular audits, and fostering a culture of cybersecurity awareness, organizations can fortify their defenses, respond effectively to incidents, and instill confidence in their AI capabilities.
Strategies for Building Trust in AI Technologies
In the world of AI, trust is key. Two vital strategies for fostering trust in AI technologies are transparency and explainability. Transparency ensures that users understand how AI systems work, while explainability provides insights into how decisions are made. Both elements help build trust by demystifying the technology and making it more accessible to users.
Additionally, user education and engagement play a crucial role in establishing trust. By involving users in the development process, educating them about AI capabilities and limitations, and seeking feedback, organizations can strengthen trust in AI technologies.
Addressing Ethical Considerations in AI Development
Developing AI ethically involves adhering to principles that prioritize fairness, accountability, and transparency. Ethical considerations in AI design require careful attention to bias identification and mitigation, ensuring that AI systems do not perpetuate discrimination or harm.
Moreover, understanding the social implications of AI technologies is essential. Considering how AI impacts privacy, autonomy, and societal structures helps developers navigate complex ethical dilemmas and create AI systems that align with broader societal values.
Compliance and Regulatory Frameworks for AI TRiSM
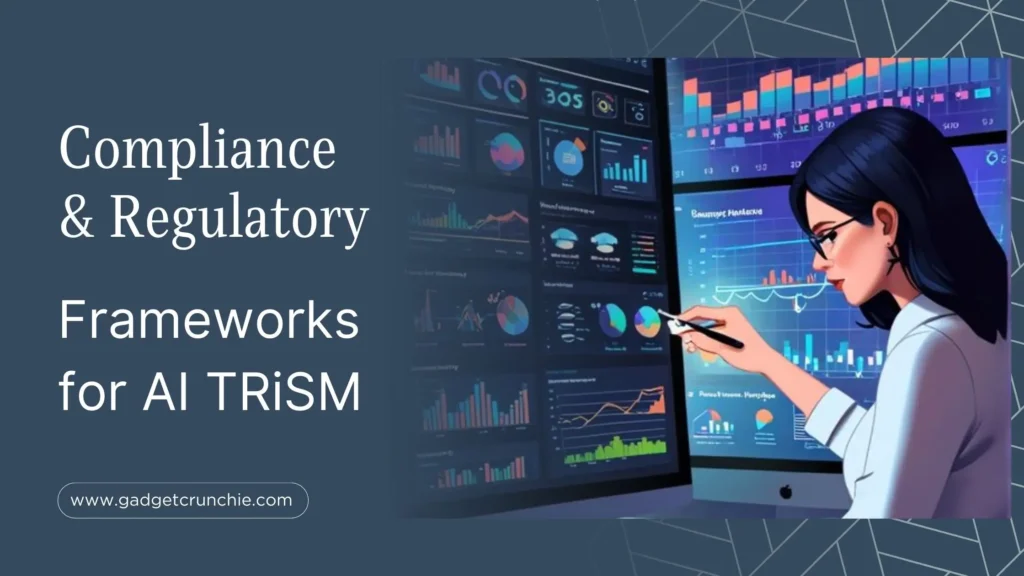
Navigating the regulatory landscape is crucial for ensuring AI trust, risk, and security management (TRiSM). Organizations must stay abreast of evolving regulations governing AI technologies to ensure compliance and mitigate risks effectively. Understanding the compliance requirements for AI deployment is essential for developing robust risk management strategies. By adhering to regulatory frameworks and industry standards, organizations can build trust in their AI systems and enhance security measures to protect sensitive data and mitigate potential risks.
Future Trends and Challenges in AI Trust, Risk, and Security Management
As AI technologies continue to evolve, new trends and challenges emerge. Emerging technologies such as autonomous systems, quantum computing, and blockchain are reshaping the AI TRiSM landscape. These technologies present both opportunities for innovation and challenges for security and risk management. Anticipating these challenges and opportunities is vital for developing proactive strategies to address potential threats and capitalize on emerging trends. By staying ahead of the curve and continuously adapting to changing technologies, organizations can enhance trust in AI systems and effectively manage risks in an ever-evolving digital landscape.In conclusion, the effective management of trust, risk, and security in the realm of artificial intelligence is essential for realizing the full potential of AI technologies while mitigating potential pitfalls. By prioritizing transparency, ethical considerations, and regulatory compliance, organizations can cultivate trust among users, minimize risks, and enhance the security of AI systems. As the field of AI continues to advance, staying vigilant, adaptable, and proactive in addressing these critical aspects of AI TRiSM will be key to fostering innovation and ensuring a sustainable and responsible AI-driven future.